OASES – Development and Demonstration of a Sustainable Open Access AU-EU Ecosystem for Energy System Modelling
Energy system modelling is the basis for the development and integration of renewable energy (RE) at local, national, regional, continental, and global levels. The resulting energy scenarios are crucial for understanding the contexts in which technologies and energy solutions need to be developed and contribute to being able to optimise future energy systems.
In the field of energy system modelling, there is a lack of holistic approaches that link all steps from the generation of RE input data, potential analysis, RE distribution, time series generation to system models. The required input data are the interfaces to the energy system model in terms of the spatial distribution of renewable energies, considering existing plants, potential areas and resource assessment, as well as the time series generation based on these.
The overall objective of the project is to develop and demonstrate a sustainable AU-EU ecosystem for energy system modelling based on open-source software and freely available data.
Fraunhofer IEE is responsible for the overall coordination of the project and is also researching the distribution of renewable energies under various boundary conditions.
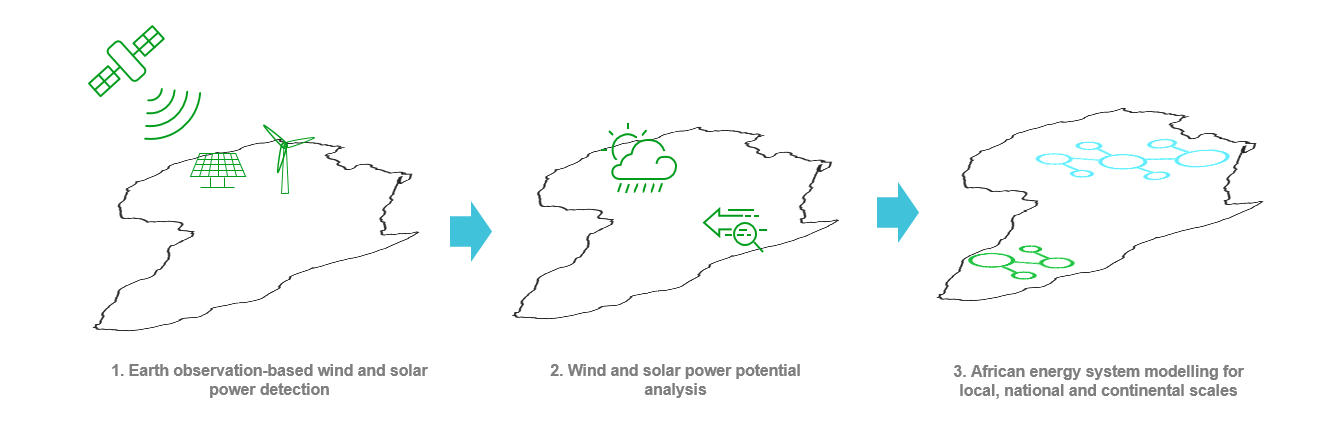